Co-Founder Taliferro
Fraudulent activities pose a significant threat to businesses and consumers alike, costing billions of dollars annually. In the fight against fraud, machine learning (ML) has emerged as a powerful tool, offering advanced techniques to detect and prevent fraudulent transactions with high accuracy.
The Power of Machine Learning in Fraud Detection
Machine learning algorithms excel at identifying patterns and anomalies in large datasets, making them well-suited for fraud detection tasks. By analyzing historical transaction data and learning from past fraudulent activities, ML models can recognize subtle indicators of fraud and flag suspicious transactions in real-time.
Key components of ML-based fraud detection include:
- Data Collection: Gathering transactional data from various sources, including payment systems, e-commerce platforms, and financial institutions.
- Feature Engineering: Extracting relevant features or variables from the data, such as transaction amounts, IP addresses, device fingerprints, and user behavior.
- Model Training: Training ML algorithms, such as logistic regression, decision trees, or neural networks, on labeled datasets to learn patterns of fraudulent behavior.
- Scoring and Classification: Assigning risk scores to transactions based on the likelihood of fraud and classifying them as either legitimate or suspicious.
- Real-Time Monitoring: Continuously monitoring transaction streams and triggering alerts or interventions for potentially fraudulent activities.
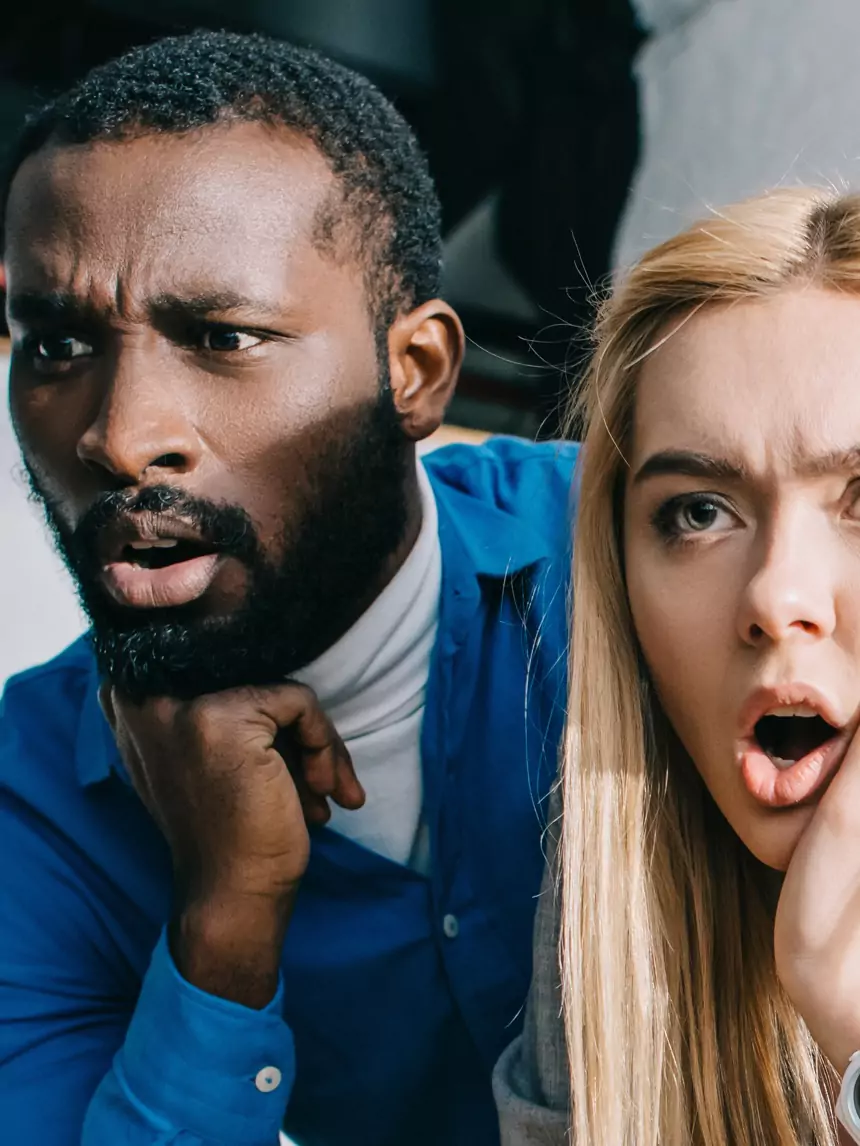
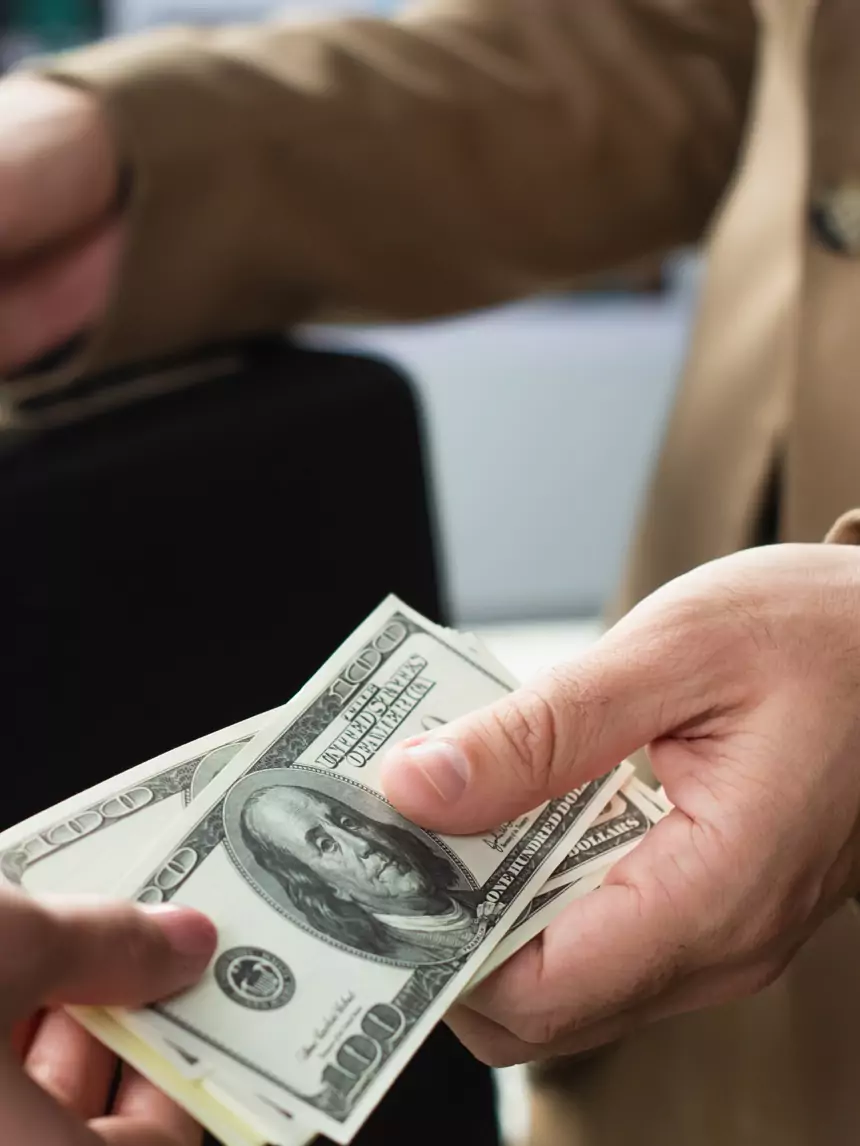
The Benefits of ML-Based Fraud Detection
ML-based fraud detection offers several advantages over traditional rule-based approaches:
- Accuracy: ML models can detect fraudulent patterns that may not be captured by predefined rules, leading to higher detection rates and fewer false positives.
- Adaptability: ML algorithms can adapt to evolving fraud tactics and patterns, ensuring effective detection in dynamic environments.
- Efficiency: Automated fraud detection processes reduce the need for manual review and intervention, saving time and resources for businesses.
- Scalability: ML-based systems can handle large volumes of transactions and scale to accommodate growth without sacrificing performance.
Real-World Applications
ML-based fraud detection has numerous applications across various industries:
- Banking and Finance: Financial institutions use ML models to detect fraudulent transactions, identity theft, and account takeovers, safeguarding assets and customer accounts.
- E-commerce: Online retailers leverage ML-based fraud detection systems to identify and prevent fraudulent orders, reducing chargebacks and protecting revenue.
- Healthcare: Healthcare providers employ ML algorithms to detect healthcare fraud, such as billing fraud and prescription fraud, minimizing financial losses and ensuring patient safety.
- Insurance: Insurance companies use ML-based fraud detection to detect fraudulent insurance claims, such as exaggerated injuries or staged accidents, mitigating fraudulent losses and maintaining pricing integrity.
The Future of Fraud Detection
As ML algorithms continue to evolve and improve, the future of fraud detection looks promising. Advancements in deep learning, anomaly detection, and unsupervised learning promise to enhance detection capabilities and reduce false positives.
However, it's essential to recognize that fraudsters are also evolving, employing sophisticated tactics to evade detection. As such, the fight against fraud requires ongoing vigilance, collaboration, and innovation to stay one step ahead of the scammers.
With machine learning as a powerful ally, businesses and consumers can better protect themselves against the ever-present threat of fraud and financial loss.